A Short History of Procedurally Generated Text
A Short History of Procedurally Generated Text
An edited version of this story has been published on tedium. This is an expanded version of the original draft.
AI is in the news a lot these days, and journalists, being writers, tend to be especially interested in computers that can write. Between OpenAI’s GPT-2 (the text-generating ‘transformer’ whose creators are releasing it a chunk at a time out of fear that it could be used for evil), Botnik Studios (the comedy collective that inspired the “we forced a bot to watch 100 hours of seinfeld” meme), and National Novel Generation Month (henceforth NaNoGenMo — a yearly challenge to write a program that writes a novel during the month of November), when it comes to writing machines, there’s a lot to write about. But if you only read about writing machines in the news, you might not realize that the current batch is at the tail end of a tradition that is very old.
A TIMELINE OF PROCEDURAL TEXT GENERATION
1230 BC: The creation of the earliest known oracle bones.
9th century BC: The creation of the I Ching, an extremely influential text for bibliomancy.
1305: The publication of the first edition of Ramon Llull’s Ars Magna, whose later editions introduced combinatorics.
1781: Court de Geblin, in his book Le Monde Primitif, claims ancient origins for tarot & ties it, for the first time, deeply to cartomancy. Tarot is now one of the most popular systems for turning random arrangements of cards into narratives, and numerous guides exist for using tarot spreads to plot books
1906: Andrey Markov describes the markov chain.
1921: Tristan Tzara publishes ‘How To Write a Dadaist Poem’, describing the cut-up technique.
1945: Claude Shannon drafts “Communication Theory of Secrecy Systems”.
1948: Claude Shannon publishes “A Mathematical Theory of Communication”.
1952: The Manchester Mark I writes love letters.
1959: William S. Burroughs starts experimenting with the cut-up technique while drafting The Naked Lunch.
1960: Oulipo founded.
1961: SAGA II’s western screenplay is broadcast on television.
1963: Marc Saporta publishes Composition No. I, an early work of ‘shuffle literature’, wherein pages are read in an arbitrary order.
1966: Joseph Wizenbaum writes ELIZA.
1969: Georges Perec’s lipogrammic novel La disparition is published.
1970: Richard & Martin Moskof publish A Shufflebook, an early work of shuffle literature.
1972: The Dissociated Press ‘travesty generator’ is described in HAKMEM.
1976: James Meehan creates TaleSpin.
1977: William S. Burroughs and Brion Gysin publish The Third Mind, which popularized cut-ups in the literary and occult sets.
1978: Georges Perec, a member of Oulipo, publishes Life: A User’s Manual, which is subject to several oulipolian constraints. Robert Grenier publishes Sentences, another early work of shuffle literature.
1983: The ‘travesty generator’ is described in Scientific American. The Policeman’s Beard is Half Constructed, a book written by ‘artificial insanity’ program Racter, is published.
1984: Source code for various travesty generators are published in BYTE. Racter is released commercially.
1997: Espen Aarseth coins the term ‘Ergodic Literature’, makes a distinction between hypertext and cybertext in his book Cybertext — Perspectives on Ergodic Literature.
2005: A paper written by SCIgen is accepted into the WMSCI conference.
2013: Darius Kazemi starts the first annual National Novel Generation Month.
2014: Eugene Goostman passes Royal Society Turing Test.
2017: Inside The Castle’s first Castle Freak remote residency produces Lonely Men Club.
2019: OpenAI releases the complete GPT-2 model.
Depending on how loosely you want to define ‘machine’ and ‘writing’, you can plausibly claim that writing machines are almost as old as writing. The earliest examples of Chinese writing we have were part of a divination method where random cracks on bone were treated as choosing from or eliminating part of a selection of pre-written text (a technique still used in composing computer-generated stories). Descriptions of forms of divination whereby random arrangements of shapes are treated as written text go back as far as we have records — today we’d call this kind of thing ‘asemic writing’ (asemic being a fancy term for ‘meaningless’), and yup, computers do that too.
But, the first recognizably systematic procedure for creating text is probably that of the medieval mystic Ramon Llull. For the second edition of his book Ars Magna (first published in 1305), he introduced the use of diagrams and spinning concentric papercraft wheels as a means of combining letters — something he claimed could show all possible truths about a subject. Much as a tarot spread produces a framework for slotting randomly chosen symbols (cards) such that any possible combination produces a statement that, given sufficient effort on the part of an interpreter, is both meaningful and true, Llull’s circles or rota do the same for domains of knowledge: one is meant to meditate on the connections revealed by these wheels.
While computer-based writing systems today tend to have more complicated rules about how often to combine letters, the basic concept of defining all possible combinations of some set of elements (a branch of mathematics now called combinatorics) looms large over AI and procedural art in general. Llull is credited with inspiring this field, through his influence upon philosopher and mathematician Gottfried von Leibniz, who also was inspired by the hexagrams of the I Ching to systematize binary arithmetic and introduce it to the west. The I Ching combines bibliomancy (divination by choosing random passages from a book) with cleromancy (divination through random numbers).
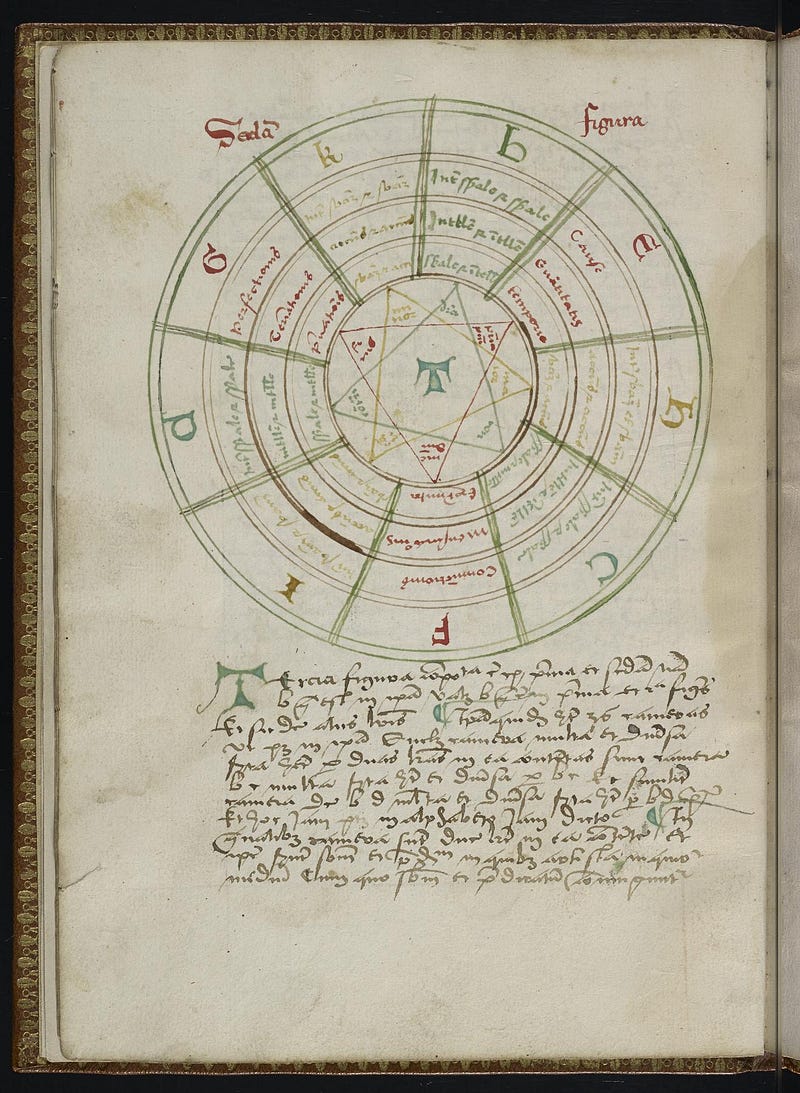
The twentieth century, though, is really when procedural literature comes into its own — and even in the modern era, the association between randomness, procedural generation, and divination persists.
Llull’s combinatorics, which had echoed through mathematics for six hundred years, got combined with statistics and in 1906 Andrey Markov published his first paper on what would later become known as the ‘Markov Chain’ — a still-popular method of making a whole sequence of events (such as an entire novel) out of observations about how often one kind of event follows another (such as how often the word ‘cows’ comes after the word ‘two’). Markov chains would become useful in other domains (for instance, they became an important part of the ‘monte carlo method’ used in the first computer simulations of hydrogen bombs), but they are most visible in the form of text generators: they are the source of the email ‘spam poetry’ you probably receive daily (an attempt to weaken automatic spam-recognition software, which looks at the same statistics about text that markov chains duplicate) and they are the basis of the nonsense-spewing ‘ebooks’ bots on twitter.
Fifteen years after Markov’s paper, the Dadaist art movement popularized another influential technique with the essay “HOW TO MAKE A DADAIST POEM”. This is known as the ‘cut up’ technique, because it involves cutting up text and rearranging it at random. It’s the basis for refrigerator poetry kits, but literary luminaries like T. S. Elliot, William S. Burroughs, and David Bowie used the method (on source text edgier than the magnetic poetry people dare use, such as negative reviews) to create some of their most groundbreaking, famous, and enduring work.
Burroughs, in his book about cutups, explains that “when you cut up the present, the future leaks out” — but it seems like when we interpret the meaningless, we see our own unknown-knowns. Divination gives us access to the parts of our intuition we might otherwise distrust, by externalizing it and attributing it to another source — and what better and more reliable source of knowledge is there than a computer, with its mythology of perfect information and perfect logic?
When computerized text generators use markov chains, a lot of the appeal comes from the information lost by the model — the discontinuities and juxtapositions created by the fact that there’s more that matters in an essay than how often two words appear next to each other — so most use of markov chains in computerized text generation are also, functionally, using the logic of the cut-up technique. That said, there are computerized cut-up generators of various varieties, some mimicking particular patterns of paper cut-ups.
David Bowie used a computer program called the verbasizer to automate a variation of the cut-up technique to produce the lyrics on his 1995 album Outside. Based on his description, I wrote a program to simulate it.
He’s no stranger to the technique — he’s been using it since the 70s.
Cut-ups can be performed by cutting out individual words or phrases, or by cutting into columns that are then rearranged. While the clip below shows Bowie cutting out individual phrases from diaries, the verbasizer simulates columnar cuts, which are then shifted up or down.
Word-based cutups are familiar to most people from their use in magnetic poetry kits. These kits are part of a long tradition of using elements of procedural art to make avant-garde work more accessible to a general audience — a tradition that is now quite lucrative.
Claude Shannon (scientific pioneer, juggling unicyclist, and inventor of the machine that shuts itself off) was thinking about markov chains in relation to literature when he came up with his concept of ‘information entropy’. When his paper on this subject was published in 1948, it launched the field of information theory, which now forms the basis of much of computing and telecommunications. The idea of information entropy is that rare combinations of things are more useful in predicting future events — the word ‘the’ is not very useful for predicting what comes after it, because nouns come after it at roughly the same rate as nouns come after all sorts of other words, whereas in english ‘et’ almost always comes before ‘cetera’. In his model, this means ‘the’ is a very low-information word, while ‘et’ is a very high-information word. In computer text generation, information theory gets used to reason about what might be interesting to a reader: a predictable text is boring, but one that is too strange can be hard to read.
Making art stranger by increasing the information in it is the goal of some of the major 20th century avant-garde art movements. Building upon Dada, Oulipo (a french ‘workshop of potential literature’ formed in 1960) took procedural generation of text by humans to the next level, inventing a catalogue of ‘constraints’ — games to play with text, either limiting what can be written in awkward ways (such as never using the letter ‘e’) or changing an existing work (such as replacing every noun with the seventh noun listed after it in the dictionary). Oulipo has been very influential on computer text generation, in part because they became active shortly after computerized generation of literature began, and in part because constraints both justify the stylistic strangeness of computer-generated text and form an interesting basis for easily-programmed filters.
In 1952, the Manchester Mark I was programmed to write love letters, but the first computerized writing machine to get a TV spot was 1961’s SAGA II, which wrote screenplays for TV westerns. SAGA II has the same philosophy of design as 1976’s TaleSpin: what Judith van Stegeren and Marlet Theune (in their paper on techniques used in NaNoGenMo) term ‘simulation’. Simulationist text generation involves creating a set of rules for a virtual world, simulating how those rules play out, and then describing the state of the world: sort of like narrating as a robot plays a video game. Simulation has high ‘narrative coherence’ — everything that happens makes sense — but tends to be quite dull, & to the extent that systems like SAGA II and TaleSpin are remembered today, it’s because bugs occasionally caused them to produce amusingly broken or nonsensical stories (what the authors of TaleSpin called ‘mis-spun tales’).
The screenplay by SAGA II (above) has quite a different feel from Sunspring, another screenplay also staged by actors (below).
This is because, while SAGA II explicitly models characters and their environment, the neural net that wrote Sunspring does not. Neural nets, like markov chains, really only pay attention to frequency of co-occurrence, although modern neural nets can do this in a very nuanced way, able to weigh not just how the immediate next word is affected but how that affects words half a sentence away, and at the same time able to invent new words by making predictions at the level of individual letters. But, because they are statistical, everything a neural net knows about the world comes down to associations in its training data — in the case of a neural net trained on text, how often certain words appear near each other.
This accounts for how dreamlike Sunspring feels. SAGA II is about people with clear goals, pursuing those goals and succeeding or failing. Characters in Sunspring come off as more complicated because they are not consistent: the neural net wasn’t able to model them well enough to give them motivations. They speak in a funny way because the neural net didn’t have enough data to know how people talk, and they make sudden shifts in subject matter because the neural net has a short attention span.
These sound like drawbacks, but Sunspring is (at least to my eyes) the more entertaining of the two films. The actors were able to turn the inconsistencies into subtext, and in turn, they allowed the audience to believe that there was a meaning behind the film — one that is necessarily more complex & interesting than the rudimentary contest SAGA produced.
In 1966, Joseph Wizenbaum wrote a simple chatbot called Eliza, and was surprised that human beings (even those who knew better) were willing to suspend disbelief & engage intellectually with this machine. This phenomenon became known as the eliza effect, & it’s here that the origins of text generation in divination once again join up with computing.
Just as a monkey typing on a typewriter will eventually produce Hamlet (assuming a monkey actually was random — it turns out they tend to sit on the keyboard, which prevents certain patterns from coming up), there is no reason why a computer-generated text cannot be something profound. But, the biggest factor in whether or not we experience that profoundness is whether or not we are open to it — whether or not we are willing to believe that words have meaning even when written by a machine, and go for whatever ride the machine is taking us on — the eliza effect is akin to suspension of disbelief in cinema or the kind of altered state of mind that goes along with religious and magical ritual, and it should not be surprising that the techniques of rogerian psychotherapy (coming as they do from the humanistic movement in psychology, with its interest in encounter therapy and other kinds of shocking reframings) would produce it in an effective way: eliza, like a tarot deck, is a mirror of the querent’s soul.
All the refinement that goes into these techniques is really aimed at decreasing the likelihood that a reader will reject the text as meaningless out of hand — and meanwhile, text generated by substantially less nuanced systems are declared as genius when attributed to William S. Burroughs.
Some text-generation techniques, therefore, attempt to evoke the eliza effect (or, as Stegren & Theune call it, ‘charity of interpretation’) directly. After all, some kinds of art simply require more work from an audience before they make any sense; if you make sure the generated art gets classified into that category, people will be more likely to attribute meaning to it. It is in this way that ‘Eugene Goostman’, a fairly rudimentary bot, ‘passed’ the Royal Society’s turing test in 2014: Eugene pretended to be a pre-teen who didn’t speak English very well, and judges attributed his inability to answer questions coherently to that rather than to his machine nature. Inattention can also be a boon to believability: as Gwern Branwen (of neural-net-anime-face-generator thisWaifuDoesNotExist.net fame) notes, OpenAI’s GPT-2 produces output that only really seems human when you’re skimming, and if you’re reading carefully, the problems can be substantial.
On the other hand, some folks are interested in computer-generated text precisely because computers cannot pass for human.
Botnik Studios uses markov chains and a predictive keyboard to bias writers in favor of particular styles, speeding up the rate at which comedians can create convincing pastiches. Since you can create a markov model of two unrelated corpora (for instance, cookbooks and the works of Nietzsche), they can create mash-ups too — so they recently released an album called Songularity featuring tracks like “Bored with this Desire to get Ripped” (written with the aid of a computer trained on Morrissey lyrics and posts from bodybuilding forums).
Award-winning author Robin Sloan attached a similar predictive text system to neural nets trained on old science fiction, while Janelle Shane, in her AI Weirdness blog, selects the most amusing material after the neural net is finished.
The publisher Inside The Castle holds an annual ‘remote residency’ for authors who are willing to use procedural generation methods to make difficult, alienating literature — notably spawning the novel Lonely Men Club, a surreal book about a time-traveling Zodiac Killer. Lonely Men Club is an interesting stylistic experiment — deliberately repetitive to the point of difficulty — and actually reading it could produce an altered state of mind; it is theory-fiction embodied in a way that an unaided human probably could not produce, and it was itself produced in an altered state (days of little sleep).
The use of automation and its function in the advancement of potential literature is important and under-studied. Machine-generated text has the capacity to be perverse in excess of human maxima, and to produce inhuman novelty, advancing the state of art. Meanwhile, commercial applications are overstated, with the exception of spam.
NaNoGenMo Entries Worth Reading Some Of
Writing 50,000 words in such a way that somebody wants to read all of them is hard, even for humans. Machines do considerably worse — a deep or close reading of a novel-length machine-authored work may constitute risk of infohazard (as MARYSUE’s output warns). That said, some entries are worth looking at (either because they’re beautiful, perverse, strange, or very well crafted). Here are some of my favorites.
Most Likely to be Mistaken for the Work of a Human Author: MARYSUE, an extremely accurate simulation of bad Star Trek fanfiction by Chris Pressey.
Text Most Likely to be Read to Completion: Annals of the Perrigues, a moody and surreal travel guide to imaginary towns by Emily Short. Annals uses a tarot-like ‘suit’ structure to produce towns with a difficult-to-pinpoint thematic consistency — suits like ‘salt’ and ‘egg’, each with its own group of attributes, objects, and modifiers.
Most Likely to be Mistaken for Moody Avant-Garde Art: Generated Detective, a comic book generated from hardboiled detective novels and flickr images, by Greg Borenstein.
Lowest Effort Entry: 50,000 Meows, a lot of meows, some longer than others, by Hugo van Kemenade.
Most Unexpectedly Compelling: Infinite Fight Scene, exactly what it says on the tin, by Filip Hracek.
The Most Beautiful Entry That Is Completely Unreadable: Seraphs, a codex of asemic writing and illustrations by Liza Daly.
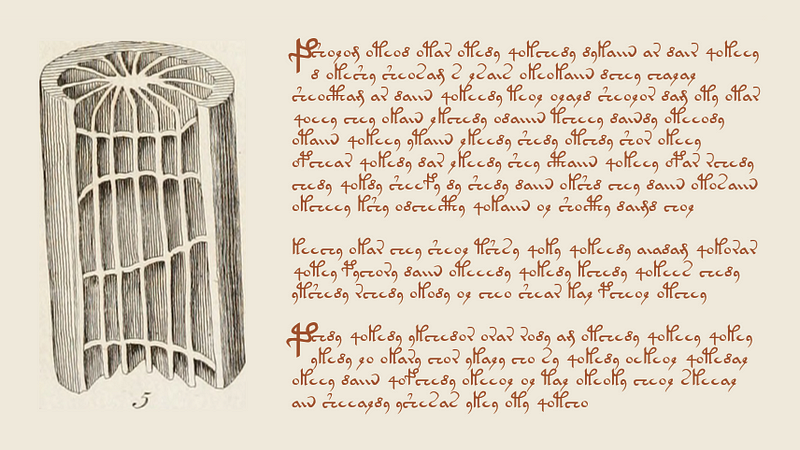
Image from Seraphs by Liza Daly, by permission
Machine-generated text isn’t limited to the world of art and entertainment.
Spammers use markov chain based ‘spam poetry’ to perform bayesian poisoning (a way of making spam filters, which rely on the same kinds of statistics that markov chains use, less effective) and template-based ‘spinners’ to send out massive numbers of slightly-reworded messages with identical meanings. The sheer scale of semantic mangling at work in spam is itself surreal.
Code to generate nonsense academic articles was written in order to identify fraudulent academic conferences and journals, which then became used by fraudulent academics as a means of padding their resume, leading to several major academic publishers using statistical methods to identify and filter out these machine-generated nonsense papers after more than a hundred of them successfully passed peer review. Somehow, actual scientists read these papers and decided they were acceptable.
The associated press is generating some of its coverage of business deals and local sports automatically, and some activists are using simple chatbots to distract twitter trolls from human targets.